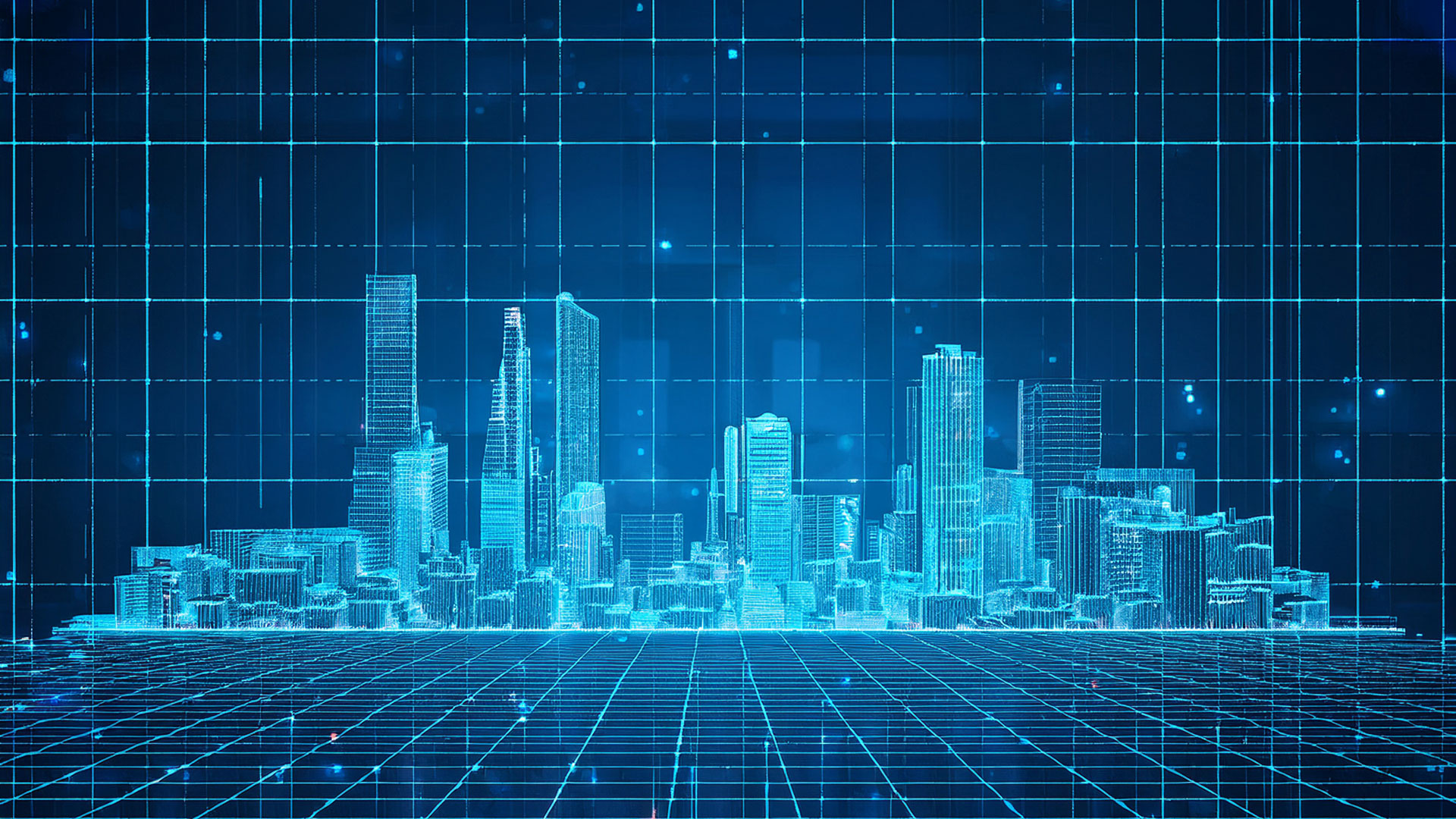
A.I.W.G. Members
Professor Maria Lonsdale (Head of School of Design)
Associate Professor Arjun Khara (A.I.W.G. Lead)
Agnieszka Rudel
Duncan Kemp
Dr Elif Sener
Dr Francisco Queiroz
Associate Professor Jeanne-Louise Moys
Joseph Gilmore
Lucy Clanfield
Associate Professor Matthew Lickiss
Professor Ningtao Mao
Dr Paul Wilson
Dr Pinar Yelmi
Rachel Hartshorne
Dr Rafiq Elmansy
Dr Raheleh Jafari
Dr Sahar Arshi
Professor Stephen Westland
Uzair Ahmad
Dr Yiting Duan
Dr Yuchan Zhang
Dr Yuri Siregar
A.I.W.G. Research
AI-101: a beginner’s guide to terms and topics
Snapshot: For many of us new to the field of artificial intelligence, understanding begins with exploration of and exposure to foundational concepts as well as core terminology. These include machine learning (ML), which enables systems to learn from data; neural networks, which mimic the structure of the human brain to process information; and algorithms, step-by-step procedures used to perform tasks for various purposes and goals.
In addition to technical terms and processes, We also explore the many ethical considerations of using AI, such as bias, fairness, transparency, and morality, alongside practical programming tools and platforms. A robust grasp of these key terms and concepts helps provide context, and serves as a critical entry point for engaging with applications and discussions around AI uses, policies, problems, and potentials. The A.I.W.G. conducts training for staff to gain a foothold and remain apprised of the newest developments in the field.
AI in XR technologies and applications
Snapshot: Integrating AI into extended reality (XR) technologies, i.e. virtual reality (VR), augmented reality (AR), and mixed reality (MR) offers exciting prospects for interactive digital experiences. AI can enhance XR experiences through the use of adaptive environments, intelligent avatars, real-time object recognition, and personalised user interactions. AI-driven natural language processing allows for a more immersive conversational experience in VR environments, while computer vision supports spatial mapping and object tracking in AR applications.
This coupling of technologies can help improve overall usability and engagement while promoting the potential for XR across various fields, including education, healthcare, entertainment, and design. Nevertheless, it also raises important considerations around data privacy, algorithmic bias, and the ethical design of human-computer interfaces in increasingly immersive ecosystems. As such, this area of research is becoming increasingly popular as these technologies make greater headway into mainstream applications.
AI in colour science and information design
Snapshot: AI algorithms analyse and generate optimised colour palettes based on perceptual models, accessibility standards, and even emotional impact, thereby supporting both aesthetic and functional outcomes. In information design, AI facilitates the automated structuring and visualisation of complex data sets to enhance clarity, engagement, and interpretability.
Machine learning techniques can also be used to personalise visual content according to user preferences and cognitive profiles. This intersection of AI with design and perception not only advances efficiency and creativity but also drives critical reflection around key areas of inclusivity, legibility, and the potential for understanding and mitigating algorithmic biases in visual representations.
AI in digital document design and fairness
Snapshot: AI features significantly in document design as well as fairness design by enabling more efficient, adaptive, and inclusive content creation processes. In document design, AI can automate layouts, typographic choices, and content structuring based on readability, user behaviour, and contextual relevance. This enhances accessibility and user engagement across platforms and formats.
In fairness design, AI is being increasingly employed to detect and mitigate biases in language, imagery, and user representation, thereby promoting equity in how information is communicated and perceived. However, the use of AI in these areas requires careful oversight, since algorithmic decisions can inadvertently reinforce existing inequalities if not transparently and ethically governed. As such, AI-driven design practices must balance automation with human-centered principles to ensure both, functionality and fairness.
Copyright and AI in sound design
Snapshot: Copyright in sound design protects original audio creations, such as music, sound effects and mixes, from unauthorised use. With AI-generated sounds matter can become more complicated. AI tools can generate sounds or assist in composing audio, but the copyright status is contingent on the extent of human creativity and input involved.
If a person significantly controls the AI’s output, then that person may hold the copyright. However, autonomous AI creations often fall into a legal grey area since current laws typically do not recognise non-human authorship. This evolving landscape is especially important for sound designers using AI in professional and commercial works.
Copyright and AI in visual design
Snapshot: Copyright in visual design typically safeguards original artworks, illustrations, and graphic compositions by granting creators exclusive rights to their uses and distribution. As such, the integration of AI into visual design processes complicates traditional notions of authorship as well as ownership.
While AI can autonomously generate complex visual outputs, current copyright frameworks generally require a human author for protections to apply. Therefore, works produced solely by AI are often not be eligible for copyright unless a human has exercised sufficient creative control over the entire process. As AI tools become more prevalent in design practices, ongoing legal and ethical discussions continue to shape the boundaries of authorship and intellectual property around generative AI.
Explainable AI: decoding the black box
Snapshot: Explainable AI (XAI) refers to approaches that help make the internal workings of AI systems — in particular, advanced and complex models like deep neural networks — more transparent and understandable to humans. AI models are often termed “black boxes” due to their opaque decision-making processes. The “thinking” employed by such models can be difficult to interpret or forecast.
Accordingly, decoding the black box involves developing tools and technical and ethical frameworks to reveal how specific inputs will influence the AI’s outputs, thereby allowing users to trace the logic behind AI-generated outcomes. Such transparency is vital not only for building trust and accountability, but equally for ensuring fairness, safety, and compliance across a wide range of applications, from healthcare and finance to law, education, governance, and creativity.
Generative AI: resources, uses, misuses
Snapshot: GenAI refers to a specific group of AI models designed to create new content such as text, images, audio, or video. GenAI achieves this by learning patterns from existing data fed to it. The technology also leverages architectures like Generative Adversarial Networks (GANs) and large language models. As such, GenAI has grown in prominence in fields ranging from creative arts and design, to scientific research and education.
However, these capabilities come at a price and present significant risks. Misuses of GenAI include production of deep-fakes, intellectual property infringement, the spread of misinformation, and the amplification of harmful biases. Without strong ethical frameworks in place, misusing generative technologies has the potential to create widespead challenges for communities, including those outside of digital realms.
Plagiarism, detection, and fair use
Snapshot: Generative AI systems can produce text, images, or code that closely resembles existing works, thereby raising concerns about unintentional and deliberate replication without appropriate attribution. Traditional plagiarism detection tools may therefore struggle to identify AI-generated content due to its novel yet derivative nature — a quality often recognisable (though more difficult to define) by academics and educators.
Furthermore, the boundaries of fair use are increasingly tested by advanced AI outputs capable of remixing and synthesising copyrighted material. As a result, institutions and governments are faced with the rising challenges of developing updated frameworks that attempt to reinstate academic and creative integrity while accommodating the evolving capabilities of AI — not an easy task by any means and as such makes for a promising area of research.
SustAInable: the triple planetary threat
Snapshot: Sustainable AI encompasses the development and deployment of AI technologies to help mitigate the environmental impacts of human activities and bring long-term balance to the ecologies in which we exist and operate. This is particularly relevant in the context of the triple planetary threat: climate change, biodiversity loss, and pollution. The energy-intensive nature of training and operating large-scale AI models contributes significantly to carbon emissions, while the production and disposal of hardware raise serious concerns around resource depletion and electronic waste accumulation.
Unchecked AI-driven practices are exacerbating environmental degradation through gross overconsumption and increasingly unsustainable automation. Addressing these challenges thus requires integrating sustainability principles into AI design, governance, and usage to align technological advancement with humankind’s planetary environmental goals. The A.I.W.G. at the School of Design has been running a series of workshops, titled SustAInable Design, with the goal of increasing awareness around resource consumption for AI technologies while also using such technologies to counter these problems.
✎
For prospective students: if you are interested in pursuing postgraduate study or research on AI and design-related disciplines, please get in touch with Dr Arjun Khara at the School of Design, University of Leeds ›
A.I.W.G. Seminars and Events
Sep 2025
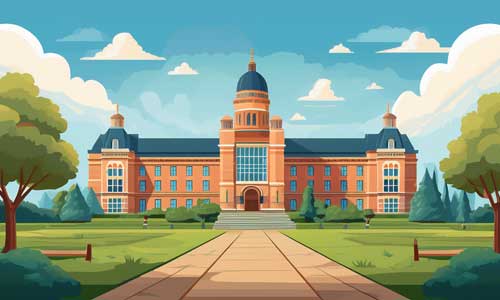
AI in Design: AI mandatory training
May 2025
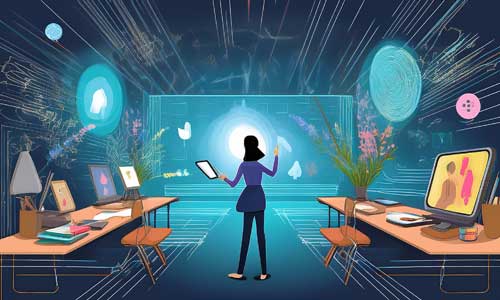
AI in Higher Education: strategies and constraints
Feb 2025
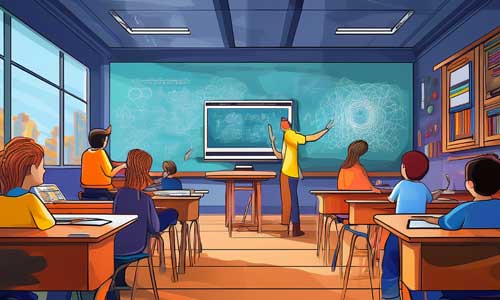
AI in Modules: implementing AI policy
Aug 2024
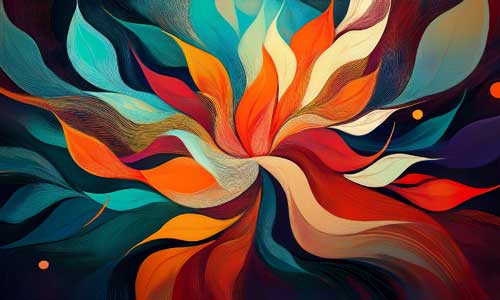
Sustainable AI: how much is it costing us, really?
Sep 2023
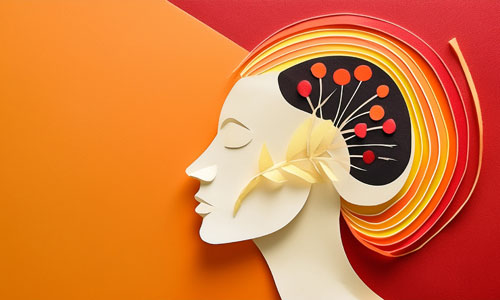
Introducing Prompts: tokens and temperature